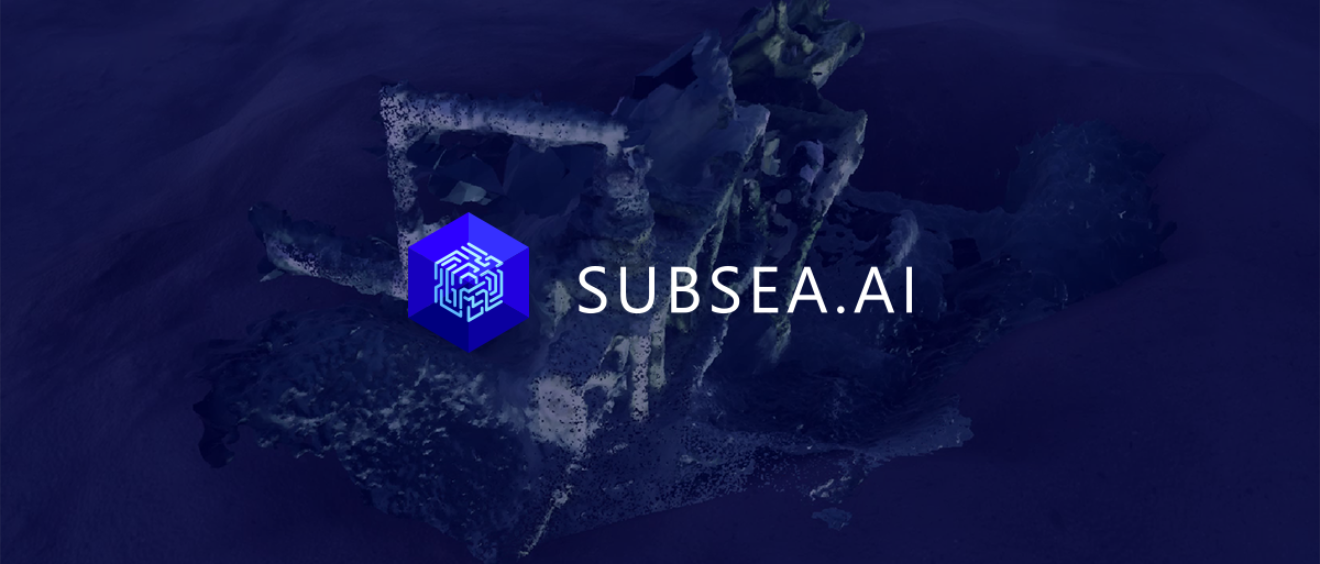
SUBSEA.AI
Artificial Intelligence
Artificial Intelligence (AI) has been lauded as the next technological revolution, right next to electricity that transformed every industry and created huge economic value. It has the potential to impact the existing healthcare, transportation, and energy industries as well as possibly create entirely new industries. Despite all this promise, AI is still at a very early stage of implementation, with few automated systems in production.
However, the technology is there to automate most of the routine tasks such as visual inspection and marine growth measurements. This represents a big opportunity for companies to be the first to adopt AI into their pipelines as this transformation will lead to improved efficiency and safety.
But, if software development is hard, AI development is even harder. That’s because AI development still faces the same challenges as software development but is further augmented by issues regarding data (quality, bias, different out-of-sample distribution, just to name a few) and the bigger difficulty of predicting project requirements and the likelihood of success. All this happens because, on one hand, there are still many open scientific questions regarding data and model requirements while, on the other hand, there is a lack of consensus as to what are the best practices for AI development. A lot of AI projects are developed without any structured process or by directly importing the software development processes into AI development. This leads to a lower success rate for AI projects.
It is well known that the most important key to success in an AI project is data. It is important to understand the data and, therefore, it is important to understand the industry that we are working in. And that is why we are launching Subsea.ai, an end-to-end AI development service for the subsea environment.
Subsea.ai
Subsea.ai is a set of services and tools designed to help in all phases of AI projects, from data storage to AI model deployment. You can choose the set of services or tools that best suit your needs, depending on the stage of development of your own AI project.
We break the process into three main stages: Data Annotation, AI Model Development, and AI Model Deployment. Each of these stages consists of several services, tools, and processes designed with the goal of developing AI applications specifically for the subsea environment, as displayed in Figure 1. These stages were defined after extensive experience and iteration in AI development for the subsea.
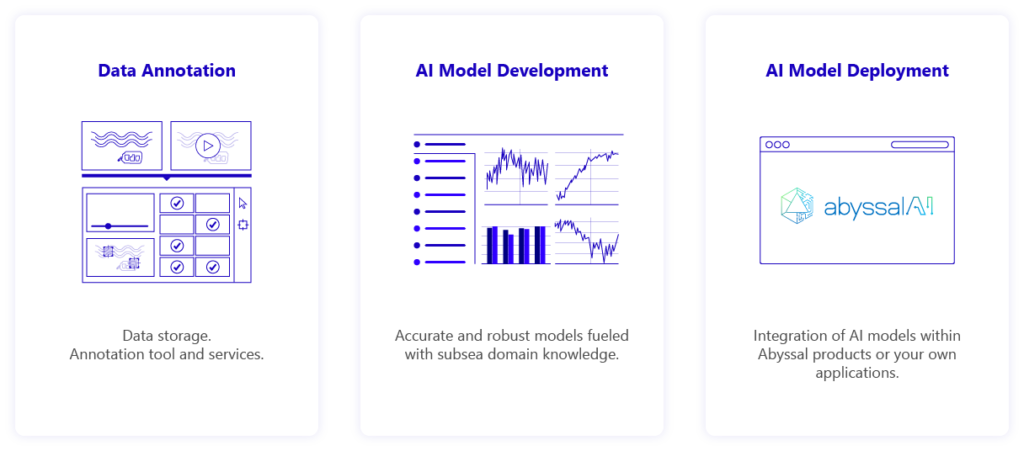
Figure 1. Subsea.ai can be divided into three main stages: data annotation, AI model development, and AI model deployment. Each of these stages is composed of a set of tools and services carefully designed with the goal of developing AI models for the subsea environment. Clients can choose all of these tools and services, or a subset of them according to their needs.
Data Annotation
All AI projects start with data and a clear task definition. Data must be stored in a place where every stakeholder (AI developers, clients, and so on) can easily access it. As data is one of the most important assets for your business, we make sure that your data is secured properly. You can define and customize the access permissions for each user and role. Not only is data stored securely, but we also make sure to anonymize the data whenever the AI task allows it, deleting anything that is not actively used within the project.
However, data by itself is not enough to develop AI models. In order to “teach” AI models, we need to provide the algorithm with input examples (that is, images of a pipeline inspection) and the correct answer for the task at hand (whether or not there is an anomaly in the pipeline). After this learning process, the AI model is able to predict if an image of a pipeline inspection displays an anomaly without any human supervision.
Therefore, the data needs to be annotated. We provide tools for you to annotate your data such as the one depicted in Figure 2 or, if you prefer, we annotate your dataset for you. Whether you need to clearly outline a particular object in an image, draw a bounding box around a fish, or simply classify the grade of severity of an anomaly in an image, we provide the best tools for you to perform those tasks on the cloud.
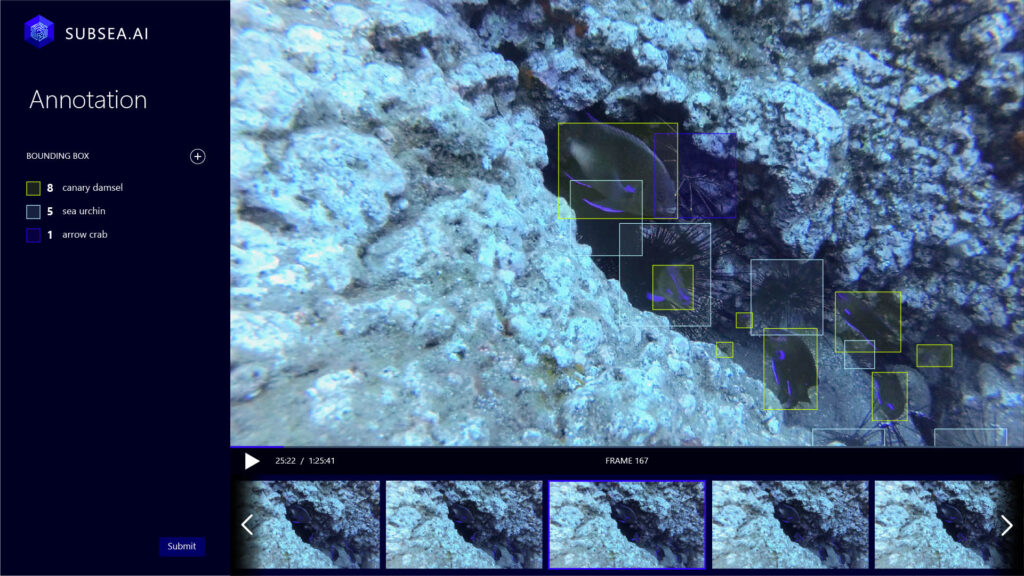
Figure 2. An example of a tool to annotate videos for a fish detection task. You can select the frames where fish are visible with the help of AI models that simplify the process.
Even though data annotation is an important step in the development of AI projects, it does not directly provide value to you. Therefore, we focus on minimizing the annotation effort, while maximizing the data annotation quality. We have developed Active Learning technology to sample the most relevant data examples for you to annotate and, in some of our previously published experiments, we have shown an 80{2beb0a36b303351603b1ea91ff18d19c17aee5e2ba0d5fe7ffa4fdb034433a0f} decrease in the data annotation effort.
Another advantage of having domain expertise is that we can use some of our existing AI models to help the user annotate data. Existing AI models can filter out low-quality examples and pre-annotated data, leaving it up to you to confirm or correct its predictions. This may have a significant impact on the usability of the annotation tools and, also, in the time spent annotating each data example.
This feature can also improve data annotation quality. The AI models can help reduce missed annotations and also standardize the annotations, as all users start from the same initial annotation prediction. Annotation quality is very important as the AI models can only learn what is in the data. If the quality of the annotations is poor, then so too will be the quality of the model. Therefore, we have a lot of processes in place to ensure the highest annotation quality. For example, the same example may be given to multiple annotators to confirm annotation variability. The platform is also constantly monitoring the annotation distribution with the goal of having a dataset that will ease the AI model optimization process while keeping the validation of the model statistically significant.
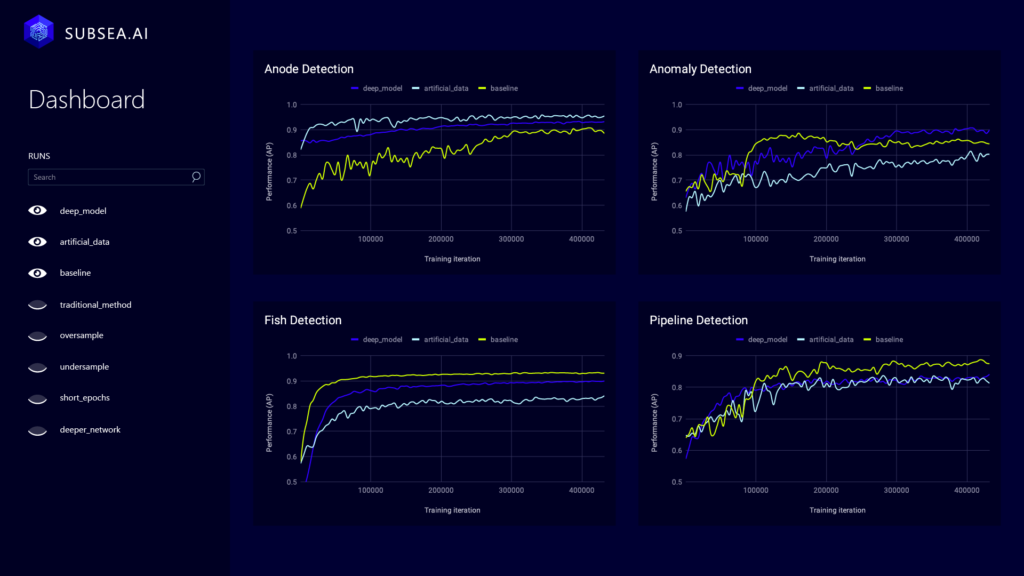
Figure 3. The performance of the AI models being developed is carefully monitored at each step. These objective metrics can help the client realize the business value of the solution.
AI Model Development
After the dataset has been annotated and its quality ascertained, we can develop the AI model. We have a team with many years of experience in the subsea domain, and a lot of AI technology already developed that can be used to reduce the risk of the AI project and achieve the best results in a timely manner. In more complex situations, when the data may be scarce or the problem may be harder, we can even make use of our Simulator to generate artificial data. The artificial data can then be used to augment the dataset with real examples and boost performance.
The performance of the AI models is also carefully monitored, as shown in Figure 3. At the end of the development process we can provide objective metrics, such as the accuracy of the AI model to make it easier to compare with human specialists, and business metrics to help the client understand the true value of the solution.
AI Model Deployment
The AI model can only provide value when it is deployed in the real world, making predictions about new data that is coming in. Depending on the needs of the client, we can integrate the AI model with our other products, such as Abyssal Offshore and Abyssal Cloud, as shown in Figure 4, to make the deployment as fast as possible. Additionally, we can help our clients integrate the results of the AI model with the solutions they currently have in place.
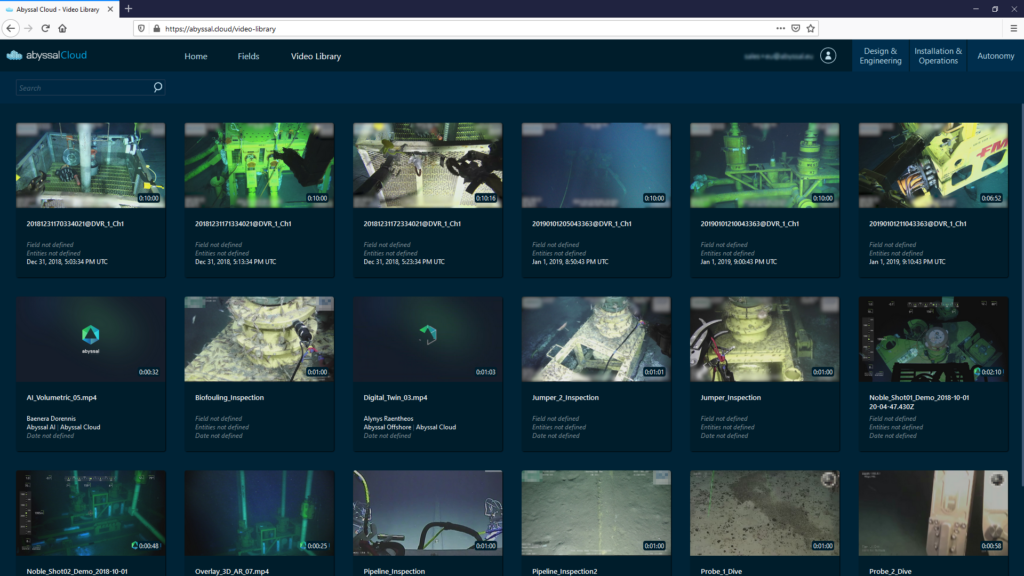
Figure 4. AI models can be deployed automatically in our products, such as Abyssal Cloud. We can also help our clients integrate the developed AI models into the solutions they already have in place.
Conclusion
There is no doubt in the industry that AI will have a tremendous impact in the future. As the implementation of AI models is still in its infancy, there is a lot of progress to be made, though hopefully in the near future. Domain expertise and good practices are key to the success of AI projects, and that is what Subsea.ai hopes to achieve.
Subsea.ai brings AI one step closer to the subsea. If you have any problem for the subsea domain and you think it can be solved using AI, don’t hesitate to contact us.
Stay tuned to ride the next wAİve of subsea automation!
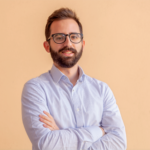
Pedro Costa
Pedro Costa holds a MSc (2015) in Informatics and Computing Engineering at the Faculty of Engineering, University of Porto. Costa started working with INESC TEC in 2014 trying to find adverse drug reactions in biological data using Machine Learning. After a brief experience in the industry, Costa came back to INESC TEC to work on medical image processing using Deep Learning methods, having published several papers in top conferences and medical imaging journals. He then spent three months working on weakly supervised deep learning methods at Carnegie Mellon University (CMU). Currently, he is Head of Research at Abyssal, working on how to improve Remotely Operated Vehicles’ operational efficiency using Machine Learning and Computer Vision techniques. He is also a PhD student at the University of Porto and keeps cooperating with CMU on several projects.